Paper on AI for equipment health state prediction at CAiSE 2021 conference
02/07/21
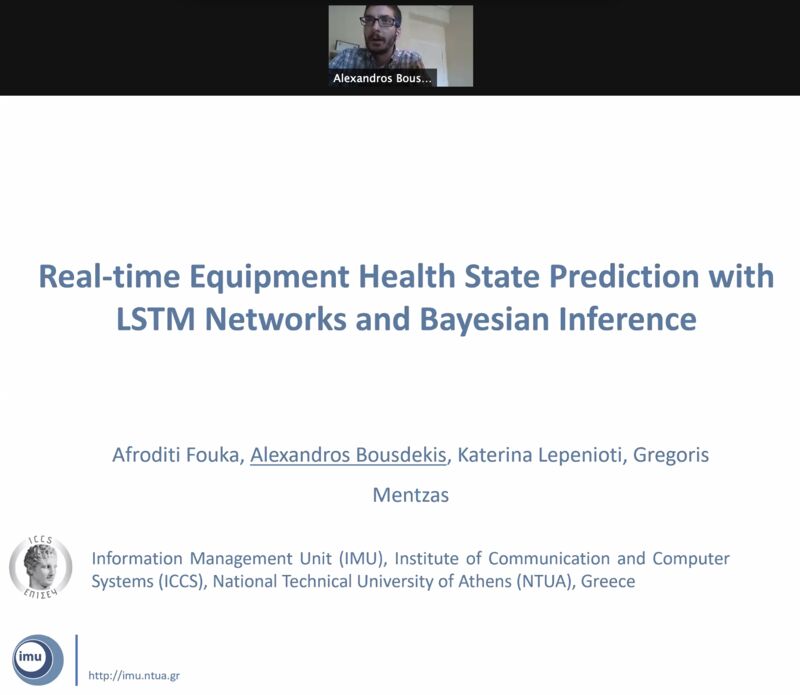
Alexandros Bousdekis presented at the CAiSE Conference on Advanced Information Systems Engineering our paper co-authored with Afroditi Fouka, Katerina Lepenioti and Gregoris Mentzas, on using state of the art Artificial Intelligence for predicting the health state of equipment in real-time.
The paper is available at: https://link.springer.com/chapter/10.1007%2F978-3-030-79022-6_13
Our paper argues that existing algorithms in predictive maintenance suffer from several limitations related to their scalability, efficiency, and reliability preventing their wide application to various industries. We propose an approach for real-time prediction of the equipment health state using time-domain features extraction, Long Short-Term Memory (LSTM) Neural Networks, and Bayesian Online Changepoint Detection (BOCD). We applied our framework to a real-life case in the steel industry and did extensive experiments that proved its effectiveness.
Research reported in the paper was co-funded by the UPTIME Unified Predictive Maintenance System and COALA Your Factory Assistant projects within ICCS – NTUA .
Recent News
New journal paper on Trustworthy AI
Our latest research publication, “Trustworthiness Optimisation Process: A Methodology [...]
Two new journal papers published on AI topics
Two new papers by IMU researchers on Trustworthy AI [...]
Presentation at Trustworthy AI conference
At the 4th conference of the TAILOR Network of Excellence [...]