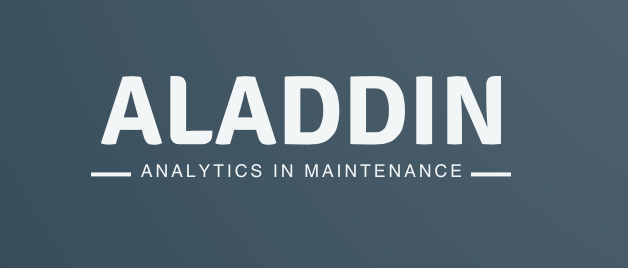
Industry 4.0 has led to an extensive use of sensors for condition monitoring, something which facilitates decision making under time constraints. Decision making in predictive maintenance indicates the phase which is triggered by sensor-driven, (near) real-time predictions (e.g. about a future failure mode) in order to generate proactive recommendations about maintenance actions and plans that eliminate or mitigate the impact of the predicted equipment failures. The P-F interval, i.e. the time between the point where it can be found out that it is failing (potential failure) and the point where it has failed (functional failure), can be seen as an opportunity window during which decision making algorithms can recommend actions with the aim to eliminate the anticipated functional failure or mitigate its effect. However, the stochastic degradation process leads to high uncertainty in (near) real-time decision making.
ALADDIN implements a prescriptive analytics approach in order to provide proactive recommendations (prescriptions) about optimal actions and plans by receiving streams of predictions about future states (e.g. failure modes for a specific asset). Moreover, it takes into account user’s preferences and expertise, that are neither quantifiable nor available through historical data, without explicitly defining them, in order to build an optimized human-machine collaboration. The ALADDIN models the decision-making process instead of the physical manufacturing process, in order to be generic and applicable to various manufacturing processes, while it is augmented by human experience and skills.
In order to enable the applicability of the ALADDIN to various settings where different kind of data may be available, two different decision methods have been implemented, a model-based one, based upon Markov Decision Process (MDP), and a model-free one, based upon Interactive Reinforcement Learning. Upon the arrival of new predictions for upcoming failures and according to the prediction’s encapsulated data, one of the two implemented decision methods is triggered. The need for two alternative decision methods relies on two main reasons: (i) To address different requirements and needs according to the manufacturing environment; and, (ii) To be applicable for different data encapsulated into the received predictions.
Key Advantages
- It brings prescriptive analytics into predictive maintenance for facilitating proactive decision making.
- It processes streams of predictions in a scalable and efficient way.
- It is able to recommend actions for both perfect and imperfect (of various degrees) maintenance.
- It combines machine intelligence with human intelligence in order to result in optimal decisions.
Application
ALADDIN was developed in the context of the H2020 UPTIME project (https://www.uptime-h2020.eu/) and was integrated in the UPTIME platform in order to address the DECIDE phase of the UPTIME framework.
For more information about the integration of the ALADDIN in the UPTIME platform, please see: https://www.youtube.com/watch?v=TyGj0BO3aJs